AI Diagnosis in the Fight Against Skin Cancer
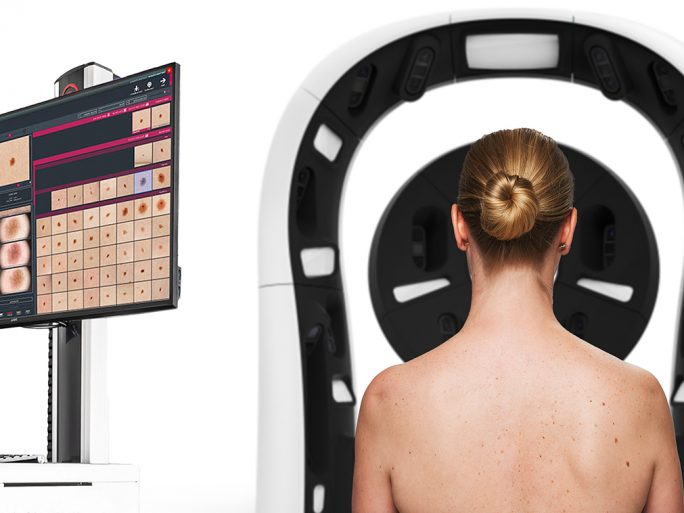
In the early detection of melanomas, a novel full-body scanner connected to an AI diagnostic platform will now provide support.
In just six minutes, it automatically examines the entire body and provides a risk assessment for each suspicious skin change. To ensure the AI used is reliable and safe, researchers at the Fraunhofer Institute for Telecommunications, Heinrich Hertz Institute (HHI), are integrating XAI techniques—explainable artificial intelligence—into the tools. Timon Meyer from Fraunhofer explains the approach:
Melanomas are responsible for 60 percent of all malignant skin tumors. Their incidence has increased significantly in recent years. They are often detected too late because current early detection methods are labor- and cost-intensive. During a full-body skin examination, dermatologists inspect each pigmented skin lesion individually for typical signs of melanoma—a time-consuming and error-prone health check.
In the EU project iToBoS, short for Intelligent Total Body Scanner for Early Detection of Melanoma, 20 project partners aim to accelerate and improve the current examination method using an AI-based full-body scanner. The goal is to increase the throughput of automated pre-assessments, optimize cancer detection, reduce the burden on medical professionals, lower patient risk, and reduce the number of unnecessary biopsies.
Developed by project partner Bosch, the iToBoS scanner automatically examines the entire body within six minutes using a cognitive AI assistant that incorporates AI models and XAI methods from Fraunhofer HHI. Medical professionals receive a risk assessment for each individual mole. The scanner is the core component of the cloud-based AI diagnostic platform also developed within the project. This platform consolidates health data from various sources such as medical records, genomic data, and in-vivo imaging. The EU is funding the initiative with €12.1 million.
Personalised Diagnostics
The scanner’s high-resolution cameras are equipped with liquid lenses that mimic the design of the human eye. Using two immiscible fluids with different refractive indices, they achieve unprecedented image quality. For highly personalized diagnostics, the captured images are integrated into the AI diagnostic platform—along with all available patient data (demographics, UV damage history, risk group, etc.)—using machine learning and the cognitive AI assistant tool.
Thanks to the scanner’s speed, not only can many patients be examined in a short time, but scans can also be repeated frequently and over extended periods. This enables effective monitoring of moles and any changes that might indicate skin cancer. Specialized AI algorithms, combined within the AI assistant, are responsible for identifying and tracking moles over time.
Verification with XAI Techniques
In sensitive application areas like medical diagnostics, AI tools must deliver completely reliable and verifiable problem-solving strategies. However, up until now, it was unclear how AI systems reached their decisions. With their project-deployed XAI methods—including patented approaches like LRP (Layer-Wise Relevance Propagation), CRP (Concept Relevance Propagation), and PCX (Prototypical Concept-based Explanations)—Fraunhofer HHI researchers are making AI predictions explainable and exposing uncertain decision-making strategies.
These methods identify and quantify a broad range of learned decision behaviors, detect undesirable patterns in massive datasets, prevent model misbehavior, and ensure the AI systems used are dependable and safe. “Until now, AI systems have been used as a black box. People trusted that they would do the right thing, which unfortunately wasn’t always the case. With our XAI methods, we’ve succeeded in making the decision-making process of AI systems understandable and overcoming their black box nature,” says Prof. Wojciech Samek, head of the Artificial Intelligence department at Fraunhofer HHI.
“Thanks to our explainable AI methods, we not only highlight suspicious moles, but also explain why they stand out. Using LRP and CRP, we visualize and interpret neural networks and other machine learning models, and we measure the impact of each input variable on the overall prediction,” adds Sebastian Lapuschkin, head of the Explainable Artificial Intelligence research group. “The ability to draw connections across huge data volumes offers the opportunity to learn more about skin cancer and individual risk potential.”
Moreover, with their R2R (Reveal to Revise) method developed within the project, the Fraunhofer HHI researchers can retrain the AI to eliminate behavioral anomalies and errors. They use information from their XAI methods to better annotate data, making the overall system more robust and reliable. The ultimate goal of the project is to develop a holistic AI model that incorporates a wide range of perspectives on patients using multimodal datasets. This is further supported by meta-datasets collected during the project in Australia (Queensland) and Spain (Barcelona), expanding the data pool by including other world regions. In the long term, the full-body scanner could also be used to detect other skin conditions such as eczema.
Project Partners
Twenty partners are developing the diagnostic system in the EU-funded iToBoS project. They are: BARCO NV, Canfield Scientific Inc., Coronis Computing SL, Fraunhofer HHI, Fundació Clínic per a la Recerca Biomèdica, IBM Israel – Science and Technology Ltd., Institute for Computer Science and Control (SZTAKI), Isahit SAS, Leibniz University Hannover, Melanoma Patient Network Europe, National Technical University of Athens, Optotune AG, Ricoh Spain IT Services SLU, Robert Bosch Española SA, Torus Actions SAS, Trilateral Research Ltd., University of Trieste, University of Queensland, University of Girona (Coordinator), V7 Ltd.