AI in Medicine: Calculating Cause and Effect
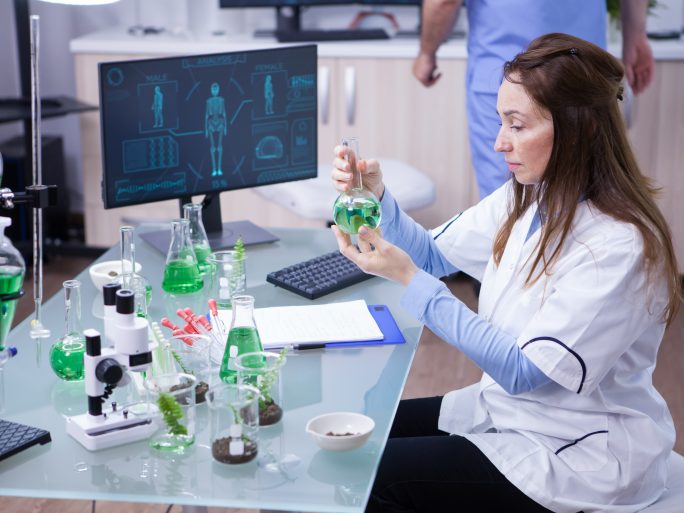
Machines can use new processes to learn not only to make predictions, but also to deal with causal relationships.
Many AI processes are being developed and tested for imaging procedures or calculating disease risks. When it comes to recognising patterns in large amounts of data, the machine can provide good service. It compares data from learned examples, draws conclusions and makes predictions.
Causal machine learning
An international team led by Professor Stefan Feuerriegel , Head of the Institute for AI in Management at LMU, is now exploring the potential of a comparatively new branch of AI for diagnostics and therapy. Can Causal Machine Learning (ML) improve treatment outcomes compared to conventional machine learning methods? The research team led by Stefan Feuerriegel believes that the effectiveness and safety of treatments can be improved in this way.
In particular, the new machine learning variant offers “a wealth of opportunities to personalize treatment strategies and thus improve the health of individual patients”, say the researchers from Munich, Cambridge and Boston. As far as machine assistance in therapy decisions is concerned, they expect a decisive leap in quality. Classical machine learning recognises patterns and discovers correlations. However, the causal principle of cause and effect generally remains hidden from the machines. They cannot address the question of why. However, many questions that arise when making treatment decisions involve causal problems.
AI recognises changes in risk due to medication
The researchers cite the example of diabetes: Classical ML would aim to predict how likely a disease is if the patient has a number of risk factors. Ideally, causal ML could be used to answer how the risk changes if the patient is given an anti-diabetes medication, i.e. a cause – the medication – has an effect. It would also be possible to assess whether a different
treatment plan would be better than the frequently administered drug metformin, for example.
However, in order to be able to estimate the effect of a – hypothetical – treatment, “the AI models have to learn to answer questions along the lines of ‘what if’,” says Jonas Schweisthal, a doctoral student in Feuerriegel’s team. “We give the machine rules for recognising the causal structure and formalising the problem correctly,” says Feuerriegel. It has to learn to recognise the effects of interventions and, as it were, to understand how consequences in reality are reflected in the computer’s data feed.
“The software we need for causal ML methods in medicine is not available out of the box.” This requires complex modeling of the respective problem, “in which AI experts and medical professionals work closely together,” says Feuerriegel.In other areas of application, such as marketing, working with causal ML has already been in the testing phase for a few years. “Our aim is to bring the methods one step closer to practical application.”