AI Strategy: Klarna eliminates 1,200 SaaS services
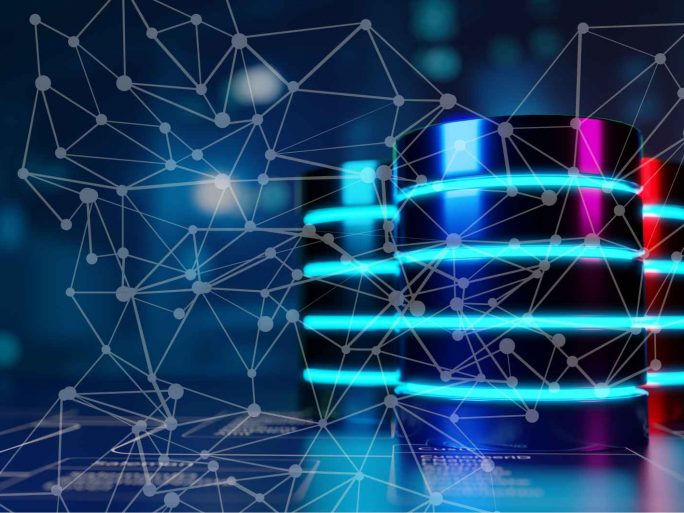
With generative AI, the era of Software as a Service is beginning to falter. The alternative is proprietary AI solutions based on LLMs and graph databases, believes Heiko Schönfelder of Neo4j.
For more than a decade, SaaS has been considered the ultimate business model for software. But now there’s increasing scrutiny as to whether this model actually meets the high expectations placed on it. One factor contributing to the potential decline of SaaS is the commodification of software.
With the rise of Artificial Intelligence and more accessible development tools, creating complex software solutions is easier than ever. Instead of paying for third-party SaaS services, companies can now develop their own customized AI solutions that are precisely tailored to their needs and can scale with their requirements.
Data quality is crucial
A current example of this technological shift is Klarna. The Swedish FinTech took a major step forward in its adoption of AI technologies last year. In autumn, it was announced that the company had replaced Salesforce CRM with its own AI system based on OpenAI, combined with the AI-native tool Cursor AI, among others. Since then, the AI-powered customer service bot has taken over the work of 700 full-time employees and is expected to save costs of 40 million US dollars annually.
In fact, Klarna has not only terminated its contract with Salesforce but has eliminated around 1,200 SaaS services in total. However, this does not signal the end of Salesforce or even SaaS, as Klarna CEO Sebastian Siemiatkowski himself explained in a post on X. According to him, the company began exploring the potential of AI and LLMs, particularly ChatGPT, early on. Klarna also reportedly “dove deep into the beautiful world of graphs” to connect various data sources, documents, and PDFs, making them AI-compatible.
Data silos vs. data context
Data quality remains a decisive factor even in the AI era, and the universal truth of data science, “garbage in, garbage out,” applies more than ever. The leading LLMs from OpenAI, Anthropic, or Google provide a good foundation. However, how powerful AI is in practice depends on the ability of the companies using it to connect and enrich the models with their own datasets. Even with the best AI model, those who can only access fragmented and inconsistent data will obtain poor results.
SaaS can contribute to these data silos in companies. In some cases, internal knowledge is distributed across a multitude of applications, from CRM and ERP systems to Kanban boards and presentation slides. Background knowledge relevant to AI, such as the context and purpose of a document, and metadata, such as users, are not automatically apparent.
Typically, SaaS applications follow their own logic, independent of the company’s overall context. To navigate the complex data network, company-specific know-how is almost always required. However, generative AI systems require the opposite: they must understand company data across different data repositories to respond and work in the context of all information.
Technology landscape with graph and AI
Graph technology has established itself as a central building block in the AI environment in recent years. Knowledge graphs, for example, represent unstructured data in such a way that LLMs can understand it as context. In the context of GraphRAG, they also serve as an additional, external data source for LLMs, thereby improving the currency, relevance, and accuracy of language models. Gartner made a similar assessment. The analyst firm expects graph technology to be used in 80 percent of data and analytics innovations by 2025 – compared to just 10 percent in 2021.
Klarna also opted for graph technology, including data modeling, ontologies, vectors, and Retrieval-Augmented Generation (RAGs). The company built a new technology landscape in which company data was consolidated and internal AI can access this knowledge. The result: Thanks to the standardized and unified knowledge base, many SaaS services are simply no longer needed.
Blueprint for AI implementation
This reorientation of AI can be used by other companies as a blueprint for AI integration. "Google search and AI are powered by a Knowledge Graph. Wikipedia's 'collected knowledge of humanity' is a Knowledge Graph. Now Klarna's organizational knowledge is also a Knowledge Graph.
In his opinion, three steps are crucial for the success of this strategy: First, an organic introduction of AI tools in the company, which is promoted and supported by the executive level but not forced. This is followed by data consolidation to free oneself from fragmented silos and map the organization's knowledge in a networked data structure – namely, a Knowledge Graph. In the final step, companies can then build AI-capable applications on this graph using GraphRAG and native AI tools.
As the heart of the next generation of GenAI stacks, Knowledge Graphs could increasingly push traditional SaaS into the background in a future shaped by Agentic AI. While many technologies are aimed at solving specific use cases, graph technology is based on fundamental principles that allow for broader application. These principles not only form the basis for initial applications in the AI field but also create the prerequisites for future developments that build on the potential of networked data.
Heiko Schönfelder
is Head of Sales DACH at Neo4j.