Digital Patient Twins: How Far Along Is the Research?
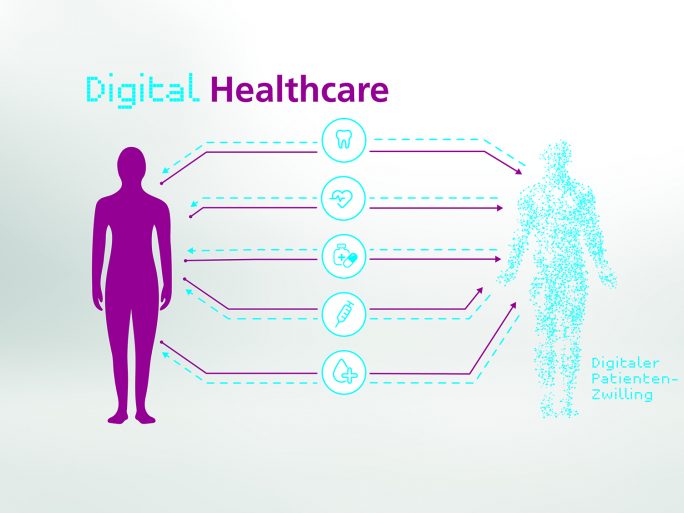
In this interview, Fraunhofer researchers discuss the progress of digital twin models in healthcare, the answers they are already providing, and what we can expect in the near future.
Creating a digital twin of a machine is a complex task—but how much more difficult is it to digitally replicate the human body? Researchers at the Fraunhofer Institute for Experimental Software Engineering (IESE) are exploring the possibilities, challenges, and potential of digital patient twins.
In the future, these digital replicas could allow medications to be tested virtually before a patient takes their first dose. In this interview, Theresa Ahrens and Jonas Marcello, co-heads of the Digital Health Engineering department at Fraunhofer IESE, explain the benefits and value digital patient twins could bring to medicine.
What is a digital patient twin?
Theresa Ahrens: A digital patient twin is essentially a highly detailed, dynamic virtual model of a biological entity. Such an advanced model can replicate cell structures, tissues, organs, or even an entire human body. Ideally, it contains all the relevant data of its real-life counterpart—the patient.
Because digital patient twins are dynamic, they can account for changes over time and simulate physiological processes. This capability makes it possible to predict bodily functions, such as how a patient might respond to certain medications.
How can digital patient twins improve medical care?
Jonas Marcello: Digital patient twins hold immense potential for a wide range of applications. For instance, they could help model metabolic processes within the body. One of the most promising opportunities in medicine is using virtual twins to assess the effects, interactions, or side effects of medications—before a patient ever takes them.
Are there parallels with machine production?
Jonas Marcello: There are similarities. Just as digital twins in manufacturing enable proactive machine maintenance, digital patient twins pave the way for predictive health monitoring.
Beyond that, they open new doors in disease prevention. By identifying early signs of illness or highlighting specific health risks, virtual twins could help patients take preventive measures in time to avoid long-term complications.
Can digital twins influence treatment methods and medical research?
Jonas Marcello: Absolutely. One key area where digital patient twins could have a major impact is clinical research. By simulating drug efficacy and dosage in a digital model beforehand, clinical trials involving real patients could become more efficient and streamlined.
Currently, only a few clinical trials worldwide involve digital patient twins, with diabetes serving as a primary model disease for this research.
What are the biggest challenges?
Theresa Ahrens: While we already have extensive knowledge of molecular mechanisms—how cells function and interact—humans are far more complex than machines. Unlike mechanical systems, the human body cannot be perfectly replicated or predicted.
As a result, digital patient twins will always face limitations, particularly in recreating the full molecular complexity of biological systems. However, even digital replicas of individual organs can provide significant value.
Which organs are best suited for digital replication?
Theresa Ahrens: Ideally, we would develop digital twin models for all organs. In some cases, we have already made significant progress.
A prime example is the digital heart twin, which is being used successfully in cardiology. This model integrates various health data and works alongside AI to analyse cardiovascular function, combining lab results, imaging scans, and other relevant information.
Another promising development is the digital lung twin, which is personalised for individual patients. In the future, doctors could use it to test different treatment and ventilation strategies in a virtual environment before applying them to a real patient—helping to ensure gentler, more effective ventilation methods.
How do you create a digital patient twin?
Jonas Marcello: Building a digital patient twin requires vast amounts of high-quality, long-term data—ideally spanning a person's entire life. Even with all the necessary data available, integrating information from various sources into a unified model is a significant technical challenge.
Data privacy is another critical concern. Who is authorised to access the data? From where? Who grants permission? Additionally, the reliability of the algorithms that power these digital models is an essential factor that must not be overlooked.
With our expertise in digital twin development, data science, and data protection, we aim to contribute to the realisation of digital patient twins in healthcare. Ultimately, this technology could drive a paradigm shift in personalised medicine, offering more precise and effective treatment options for patients.