Facts, Not Fiction: What to Do About AI Hallucinations and Bias?
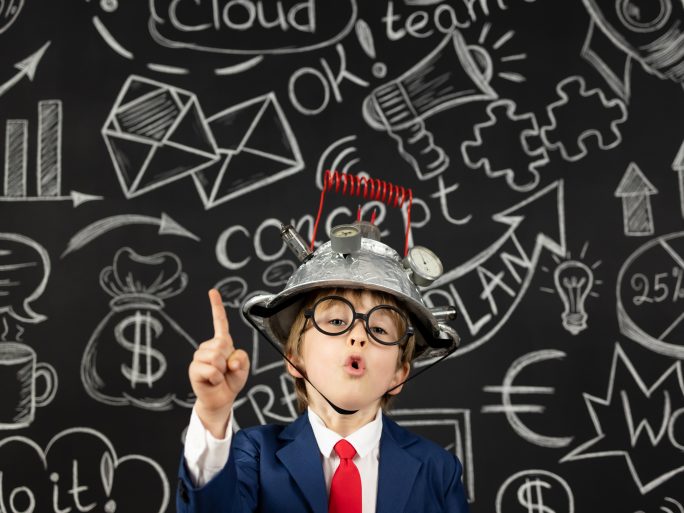
Generative AI can be wrong or deliver biased results. Here are measures that companies can take to minimize the risk of hallucinations and bias.
As versatile and powerful as AI models have become, their outputs are not always correct. They may provide inaccurate information or make unfair, discriminatory decisions.
“Responsible use of AI means not blindly trusting the output and involving a human, especially in safety- and business-critical areas. In addition to their experience and expertise, humans can also use common sense to evaluate the AI’s content and correct it if necessary,” says Jan Koch, AI Business Development Manager DACH at Dell Technologies.
The reasons for errors, hallucinations, and bias are multifaceted and range from unsuitable AI models and inferior training data to manipulation of the AI itself. Dell Technologies explains what companies can do to make their AI applications more reliable, fair, and trustworthy.
Selecting Suitable AI Models
Although large language models (LLMs), which form the basis of many GenAI applications, are trained with vast amounts of data, they are not omniscient. Nevertheless, they always strive to provide a plausible-sounding answer, even if they have to make it up. With smaller, domain-specific models, the risk of such hallucinations is significantly lower. While these models may not have the same breadth of knowledge as LLMs, they possess more specialized knowledge in a specific area. Companies should therefore choose a model that suits the respective use case, rather than trying to cover all use cases with one LLM. It also makes sense to opt for a European or open-source model, as it is easier to understand the data and rules used to train them. This makes it easier to recognize knowledge gaps and incorrect results.
Feeding AI with Internal Data
In order to provide good answers, AI models require not only the knowledge they have been trained on in advance, but also internal information from the company that fits the respective use case. This information can be integrated into the model through fine-tuning, further reducing the risk of hallucinations. Additionally, the information can be made available to the model via Retrieval-Augmented Generation (RAG), where the model uses its generative capabilities to obtain knowledge from integrated databases and documents. Both approaches can be used in combination: fine-tuning ensures that the model better understands queries and formulates answers professionally, while RAG provides a database that is always up-to-date.
Ensure High Data Quality
If companies train their own AI models or fine-tune existing ones, they must use balanced, high-quality data. Otherwise, the model cannot generate correct, fair, and unbiased answers. It is often enough for outdated or redundant training data to impair a model. For example, old data that no longer meets social standards can lead to discriminatory AI output or perpetuate stereotypes. Redundant information can overemphasize certain topics, facts, and opinions. Modern data management, which allows structured data to be accessed uniformly across all systems and storage locations, makes it easier to check, cleanse, and flexibly provide data for different AI models.
Providing Answers with Sources
If the AI model obtains its knowledge via RAG from internal databases and file shares, companies can design the output of their GenAI applications to include source information. This allows employees to verify the answers, for example, on a random basis or if the answers seem implausible. However, employees need training to formulate queries effectively, scrutinize results, and verify them when in doubt. They should also gain practical experience quickly, with more experienced colleagues available to provide support when needed.
Setting Guidelines for AI
Appropriately formulated prompts can, in some cases, cause AI models to use inappropriate language, disclose confidential data, or generate dangerous content such as malware code. Companies should prevent this with guardrails—protective mechanisms that check all input and output, ensuring that the AI does not process off-topic queries or known jailbreaking prompts, and filters out personal data, hate speech, and offensive language.
Prevent Manipulation of Training Data
Deliberate manipulation of training data can lead to incorrect, inaccurate, unfair, or discriminatory outputs from the AI. Recognizing and rectifying these manipulations is extremely difficult and costly. It’s better to protect the training material with proactive security measures. In addition to basic security practices like secure passwords and multi-factor authentication, this includes modern security concepts like Zero Trust. These methods prevent unauthorized persons from manipulating data by assigning minimal authorizations and consistently verifying all access. Additionally, advanced security and development tools help detect unusual access patterns and changes to the data, as well as fluctuations in the accuracy of AI models.
Carry Out Risk Assessments for AI Decisions
All of the above measures reduce the risk of AI errors. However, there are areas where even a low risk is unacceptable, such as in sensitive areas of people's lives or safety-critical tasks. Companies should therefore conduct a risk assessment and clarify the consequences of incorrect or unfair answers and decisions. This allows them to determine in which areas AI can operate largely autonomously and where human employees need to review and, if necessary, adapt the generated content. Control mechanisms must be implemented, particularly in security- and business-risk areas, with humans making the final decisions.