MestreLab: “Human Experience and Artificial Intelligence have different Limitations”.
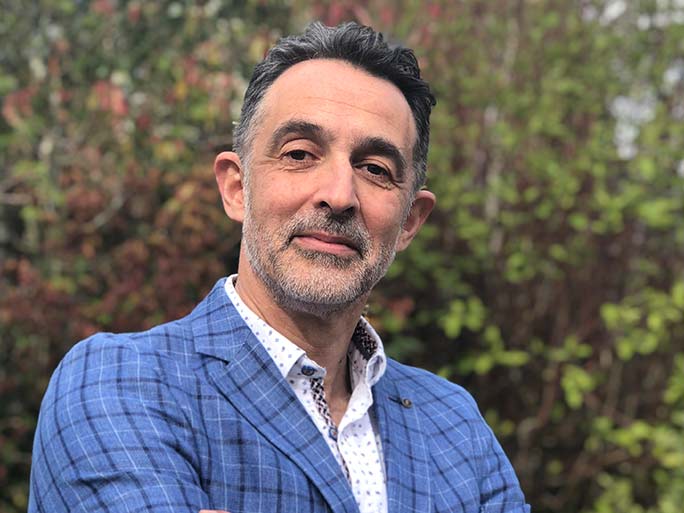
We interviewed Santiago Domínguez, CEO of Mestrelab Research about the implementation of Artificial Intelligence in medical and food solutions.
Mestrelab Research S.L. is a company that emerged as a thesis project in the Department of Organic Chemistry at the University of Santiago de Compostela. Initially, they faced a problem in the processing of data obtained by nuclear magnetic resonance, as the instruments required considerable time to acquire the data and then process it on the instrument’s computer. This limited efficiency and the ability to acquire more experiments.
Javier Sardina, one of the co-founders of Mestrelab Research S.L., proposed the idea of developing software that would allow the data to be processed outside the instrument, on a Unix platform, as the processing software available at the time only worked on Unix. Carlos Cobas, the second founder and chemical programmer, was in charge of developing this software, which eventually became his thesis project.
Subsequently, Javier and Carlos started to put the software online so that other labs could download and test it. This allowed the software to gain popularity and receive contributions from different labs around the world. At the time, the software was free.
Eventually, the possibility of creating a spin-off from the University of Santiago arose, coinciding with the start of the UniEmprende programme, the university’s first spin-off programme. At that time, in 2003, Santiago Domínguez, CEO of Mestrelab Research S.L. and third partner joined the project, and they decided to give a commercial focus to the project in order to take it to the market.
In 2004, they officially founded the company. They started working from a flat with two laptops, adopting the remote working approach that is now so common. At the time, one of the partners lived in England, as they had better access to the UK market from there. The transition from offering the software for free to charging for it was not easy, but they managed to gain traction and the company started to grow.
Today, Mestrelab Research S.L. not only offers support for MRI, but also covers various laboratory techniques, such as liquid chromatography, gas chromatography, optical spectroscopy, among others. Its main focus remains to enable scientists to extract the highest possible quality and quantity of information from experimental data, using signal processing algorithms and leveraging artificial intelligence for interpretation and analysis. Today, Mestrelab Research S.L. has more than 80 employees and continues to evolve its history.
Research sector
– How is the research sector doing in Spain and Europe and if you want to compare it with the United States or other regions of the world?
Mainly, I have a perspective on Spain, Europe, and the UK. In terms of the state of the sector, it is clear that in the US there is much more R&D funding. However, it is important to distinguish between research in public and private institutions. On the private side, I think the problems are similar, but in public and academic institutions, as well as research centres, the US has much more funding for research, as do other countries such as Korea and Japan. This is reflected in the percentage of GDP that these countries spend on research.
It is true that in the US, academia has a successful model of private funding through grants and the exploitation of patents at large universities, among others. In Europe, we have a disadvantage in this respect, as we are less well funded, but we also have the advantage of being able to do more with fewer resources. In the end, necessity sharpens ingenuity. From my perspective, it is easier to get funding for projects in the United States because of their greater funding, which allows them to be more competitive. However, competitive projects in Europe, especially in Spain, tend to be of high quality.
In Spain, for example, a lot of quality science is generated in our field, which is the life sciences. What has not been done so well in the past is to transfer that science to commercial or technology transfer initiatives. Something similar happens in industry. When you launch a company or a start-up in Europe or in the US, which involves a significant research component and therefore a considerable initial investment to move the project forward, it is much easier to fund it in the US. Generally, funding rounds are much larger, allowing more capital to be raised, speeding up the process, and increasing the chances of success.
It is true that public capital in Europe is currently filling this gap to some extent, through funds such as Next Generation and other forms of support, grants, and loans provided by public administrations. These funds cover the initial transfer phase and, in some cases, continue to support the company until it becomes sustainable. This is something that is not necessary in the US, as private equity understands the risk and is willing to invest much larger amounts.
Investment Funds
– In November 2022, Bio & Tech Smart Capital announced the closing of its first fundraising with more than 5 million euros of private capital. What types of projects are you going to finance with that budget or what idea do you have of how you are going to allocate it?
The partners of MestreLab, Javier, Carlos, and I, have created an investment instrument called Sémola Technology Ventures. As the name suggests, our objective is to finance the first phase of companies that have just left university and need that initial capital to become relevant and sustainable business projects.
Bio & Tech Smart Capital is a venture capital fund that I have personally created in collaboration with the Zendal Group and another Galician venture capital firm called Noso Capital. Our goal is to raise 50 million euros: the first closing was done with five million euros and the total target of the fund is 50 million euros. This 50 million will be used to invest in companies at a slightly later stage, i.e. companies that are starting to get commercial traction, with the aim of helping them. These are mostly technology companies related to life sciences, such as biotechnology, medical devices, diagnostics, and also digitalisation applied to foodtech, medtech, and adtech, i.e. agricultural technology, food, and medical technology.
Our intention is to accelerate these projects to become internationally competitive and to look for opportunities in international markets. As a venture capital fund, our goal is to generate returns for our investors through the sale of the company or its IPO. For most of these projects, the ultimate goal is likely to be a listing on the Nasdaq, although in many cases sales could also be made along the way.
In addition, we aim to boost the sector in Galicia with the financing we provide, which is likely to lead to additional rounds involving other funds to fill the financing need and enable the rapid growth and expansion of these projects. We do not want all the big technology projects to be developed only in the United States, as was the case in the last industrial revolution. Our goal is that, given that excellent life sciences research is being produced, as in Spain and Europe in general, internationally competitive companies will also be created and that opportunity will be spread a little bit.
Artificial Intelligence
– How does image processing through artificial intelligence work and how is it used in the diagnosis and treatment of neurodegenerative diseases?
Actually, the value of our work lies in medical image processing. The diagnosis of neurodegenerative diseases through medical imaging is established and has traditionally been performed by specialists such as radiologists or neurologists, sometimes in collaboration. However, this approach presents difficulties and biases in human interpretation. In addition, when reviewing numerous images, humans can become tired, which can lead to errors.
This is where QubioTech Health comes in, using artificial intelligence algorithms based on machine learning and deep learning techniques. The software is trained on a wide variety of medical images covering different diseases and different stages of disease progression. Based on this training, the software is able to identify problems and make diagnoses.
However, at this time we do not see QubioTech Health’s technology as a complete replacement for diagnosis by medical professionals. Instead, we see it as a support tool for radiologists and neurologists. When a specialist reviews numerous images, 10% of them may not be entirely clear due to fatigue or other reasons. The software provides a second opinion, forcing the specialist to review those images again and significantly reducing medical errors.
While we are working towards full automation, we believe there is still work to be done both in strengthening technological capacity and in building the confidence of medical professionals. There is legal liability if a doctor makes a mistake, but who takes responsibility if artificial intelligence makes a mistake? Therefore, at this point, QubioTech Health is positioned as a support tool. The tool is very effective, has obtained excellent results and is already on the market, collaborating with several hospitals and medical groups.
The feedback we receive is very positive, it is clear that it adds value. With the funding provided by Sémola and another investor, our goal is to accelerate growth and increase commercial capacity, especially internationally. While we have been successful in selling to hospitals in Spain, this is not only a problem faced by hospitals and doctors in our country, but a global challenge.
– Do you mean that artificial intelligence, for the moment, requires continuous review even as you seek to automate it?
In our case, we argue that it does. It might not, but we position our project as a support tool. Although the quality is already good enough to fully automate it, there are still aspects that are not fully resolved. Therefore, we approached it that way at the beginning.
Emerging technologies
– Are you considering introducing other emerging technologies? For example, have you considered the use of metaverse tools for the study of 3D images instead of the traditional 2D flat images?
As for virtual reality, it is not something we have explored specifically for this particular application. However, we have seen its use in some of the applications in which MestreLab employs artificial intelligence, especially in targeted therapies.
In this context, it is a three-dimensional problem in which you analyse whether a treatment, which is a molecule in three dimensions in a medium such as plasma or a liquid, fits topographically to the part of the protein with which it is intended to interact. There are many opportunities here in terms of 3D simulation, virtual reality, among others.
In the field of neurodegenerative diagnostics, we already work with 3D imaging, because what you get is a two-dimensional map of the brain, with colours representing intensities. This allows us to obtain a kind of relief, which our software understands. It may not be necessary to have the actual 3D map, as the software is more sensitive to colour and intensity, which provides information about the three-dimensional relief more accurately than the human eye. That is one of the advantages the software offers: the ability to interpret colour and intensity to obtain information about the 3D relief.
Research Centre
– Question: In December 2022 you announced the creation of a research centre. As far as the funding part is concerned, I understand that it is 9.2 million, of which about 50% is provided by the Xunta de Galicia. Could you tell me a bit about how the creation of this centre is going?
The budget for the Research Centre is 9.8 million and the Xunta is providing 4.2 million for the project. We have already started working on the creation of the centre. We have acquired the building and have started work. Our goal is to finish the project in the summer of 2024, so we have about a year left.
We are currently working on the construction and adaptation of the building. It is a historic industrial building located in Santiago de Compostela, which was abandoned for 25 years and therefore requires major restoration work. We are very excited about this project, not only because of the opportunity to accelerate our R&D, but also because of the recovery of this architecturally valuable building. It is a building that was difficult to use for private projects due to licensing restrictions and its location in the centre of Santiago, where hotels and restaurants are usually located. Industrial buildings are not suitable for this type of business.
Normally, industrial companies are established in industrial estates with lower costs and access to outdoor facilities. In our case, the location is perfect, as it is right next to the university campus known as the Campus Vida of the University of Santiago, one of the leading life sciences campuses in Europe. Literally, we cross the street and we are at the Chemical Research Centre; we cross in another direction and we arrive at the Medical Research Centre. This proximity is ideal. It was difficult to find a company that needed this facility and that would fit the needs of the company, but it has come about because of the focus we have on R&D.
– This centre will open up new lines of research in the field of biotechnology in disciplines such as artificial intelligence, advanced algorithms and scientific data management for the acceleration of research and development processes. What projects have you already planned, or are you going to wait for them to open?
We already have projects in the pipeline. They are all MestreLab’s own projects and, in fact, what is happening is that we are already working on these lines, but we need to accelerate and increase our capacity considerably. We are focusing especially on accelerating research and development of new materials, including drugs, materials and food. We are focusing on research and development in the biotechnology, biopharmaceutical, chemical and food industries.
To achieve this, our aim is to automate as much as possible all processes within R&D. Therefore, there are a number of projects that will help us to achieve this. For example, the automation of the evaluation of laboratory data. As I mentioned at the beginning, we used to face the problem of people spending an hour in front of a spectrum trying to understand what it was telling them and blocking the instrument. Today, that problem no longer exists because they do that analysis on their own laptop, at home or wherever.
However, a lot of time is still spent on the interpretation of this data. This is a complex problem when it comes to using artificial intelligence, but we believe it is a problem that can be solved. What we would achieve is to free up 40-50% of researchers’ time on these tasks so that they can focus on more value-added activities, such as drug design, rather than having to spend time deciding whether the product of a reaction is the desired one or whether it has impurities that can be used as animal or human models.
Our aim is to speed up and eliminate all these tasks that do not add the most value. In addition, we seek to contribute to the design phase of new drugs, taking advantage of artificial intelligence to generate ideas and explore much broader fields of research. Human expertise and artificial intelligence have different limitations. We have seen and experienced certain things that condition our capacity for invention, but artificial intelligence has had access to what is in my mind, your mind and the minds of many other people. At that level, it can bring a lot to the table. So all the projects are aligned in this direction.
– Is there any differentiation between sectors in terms of the application of artificial intelligence? For example, between the development of pharmaceuticals, medical products and the food sector where you also focus on.
Yes, to some extent there are differences. In the case of pharmaceuticals, for example, you are working with chemical molecules, which brings with it a number of additional challenges. You need to be able to represent and understand the molecules. You cannot teach an artificial intelligence to generate molecules without first understanding their nature, as they are completely different entities from an image.
In the field of food, on the other hand, the problem tends to be related to mixtures, which is a completely different challenge. However, an adequate representation and understanding of artificial intelligence is also required for its development. Each sector has specific issues, but they share a common technology, such as machine learning and deep learning, which rely on the processing of large volumes of data for training.
Separate datasets are generally used to assess the quality of artificial intelligence. Furthermore, in the field of biotechnology, one works with proteins, monoclonal antibodies and DNA, which are much larger entities. These molecules cannot be represented in the same way as a smaller molecule, as their three-dimensional structure is of greater importance. This adds additional challenges, as often part of the molecule’s structure is known, but not all of it, requiring models to take into account the uncertainty of as yet uncharacterised parts.
Relationship with pharmaceuticals
– How do you understand the relationship between MestreLab and pharmaceutical companies when it comes to developing artificial intelligence for the creation of new drugs or food products?
We understand them as partners. In the end, we are very specialised in certain parts of the process in which pharmaceutical companies normally do not get involved. That is, we focus on the processing of analytical data from laboratory instrumentation, which is a specific area of expertise for us. Although there are other companies in the world and there are competitors, it is generally not the pharmaceutical companies, but our customers.
They provide us with data and collaborate in the development, as we need industry-relevant data to build models, for example. They test our developments and see their usefulness, which generates a continuous feedback loop where we improve our tools with their collaboration and funding. By purchasing products, our research and development (R&D) is funded.
In some areas, such as drug design, there is some overlap, as the pharmaceutical industries themselves have groups working on these technologies. However, what we bring to the table complements their efforts. Because we work with different datasets, we broaden the field of knowledge. Pharmaceuticals are experts in their own areas of expertise, which are not the same in every company.
On the other hand, we also contribute to this global competence, as we receive data from academic institutions that pharmaceutical companies do not have access to. In the case of artificial intelligence, it’s almost a “the more the merrier”, as having a greater variety and quantity of data makes our models more powerful and more capable of covering a wider range of solutions.
– By having access to the projects developed by the different pharmaceutical companies, can you have a general idea of the needs of all these companies, so you have a fairly rich know-how?
Yes, we have a very high level of know-how. It is true that, in general, in the case of pharmaceuticals, we do not usually have access to a lot of their data, as it is highly confidential. However, in the case of academic institutions, that is where we bring our knowledge. If you look at it, 80% of chemical experimentation and research today is done in academic institutions and is funded by public capital and public administrations. These data are easier to share, as their purpose is to be published and used. It is precisely these data that contribute greatly to our technology.
So we have a very broad and less constrained know-how from that point of view. That is what we bring to the equation. And it’s not just us, there are also a lot of people working on prevention and artificial intelligence applied to drug design and development, including academic groups, startups and large companies.
In addition, our team includes people from industry, and many of the scientists leading our development have worked in companies such as Astrazeneca, Lilly, CSK and others. This allows us to bring that knowledge together and understand what is the next product our customers need.