Telecoms Move Towards Autonomous Networks
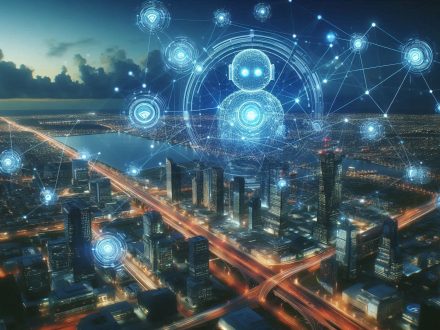
The rise of AI is sparking interest in autonomous telecommunications networks. We look into the benefits, the risks and when they will become a reality.
There are few technological advances with the impact artificial intelligence (AI) is having. This technology seems to have no limits, uncovering countless new use cases almost every day.
AI also offers a wealth of applications in the telecommunications sector, most notably developing autonomous networks. ‘Autonomous networks, based on AI technology, transcend mere automation by offering a smarter, more resilient and user-centric digital experience,’ says Claudia Muñiz, Global Head of Sales, Cognitive Network Solutions, Ericsson.
‘This enables network operators to optimise operational efficiency, enable new monetisation channels, minimise human need and maximise resource usage. Through the use of intents, formal statements that express operational objectives, autonomous networks enable proactive network management, accelerating service provision. In addition, their highly scalable and flexible architecture dynamically adapts to variations in demand and new business requirements,’ he specifies.
Juan José Marfil, director of Network, IT and TV at Telefónica España, points out that autonomous networks introduce ‘enormous advantages’ for operators, from greater agility by reducing maintenance, evolution and incident resolution times, to the reduction of errors derived from manual tasks’.
‘Telefónica has been introducing automation mechanisms in its networks for years, achieving milestones such as eliminating up to 80% of manual tasks in certain processes, thereby reducing errors by more than 60%; or reducing the deployment time of new software by 70%, achieving much more agile innovation and renewal cycles,’ he explains.
‘In addition, this automation allows optimisation of network operations related to traffic management, more efficient and secure implementation of certain processes or preventive maintenance of the network,’ he adds.
Claudia Fuentes, member of Aotec’s board of directors and CEO of TM Digital Granada, points out that AI makes it possible to ‘anticipate the detection of faults and carry out proactive maintenance’, thus reducing incidents and optimising costs, as well as ‘improving real-time adjustments to traffic variations in the network and reorganising traffic in the event of a fault’. ‘This will also be an advantage for users, who will notice an improvement in their quality of service,’ he stresses.
From the customer’s point of view, Muñiz stresses that ‘automated networks guarantee higher reliability and quality of service’. ‘The AI-powered advanced analytics of these networks allows for highly personalised services, predictive recommendations and proactive support.
For example, AI-powered automated networks can further personalise customer experience by being able to detect customer needs and deliver network resources based on their needs. ‘AI can analyse user behaviour and optimise the connection according to their specific needs. In this way, automatic adjustments are made to the network to improve the experience depending on the type of use (gaming, remote work, etc.), improving browsing speed and lowering latency,’ says Miguel Sánchez, CEO of DigitalES, the Spanish Association for Digitalisation, which represents the main companies in the technology and telecommunications sector.
This also inaugurates new business opportunities. ‘Operators can find new revenue streams through advanced automation, improving service offerings to their customers,’ says Fernando Rionegro, Market Leader Telefónica and South & Central Europe, Nokia Cloud & Network Services.
On the other hand, the Ericsson expert points out that ‘security and reliability are strengthened by continuous monitoring of traffic patterns with AI, facilitating the detection of fraud and cybersecurity threats, and implementing mitigation measures in real time’.
Sanchez also highlights that ‘AI makes it possible to improve security by introducing more levels of automatic protection against fraud, DDoS attacks and data breaches’. ‘This results in a benefit for users, who in turn experience a reduction in service interruptions and outages thanks to the self-regulation of the network.
Where do we stand?
Zigor Gaubeca, CIO of Grupo Aire, points out that there are several essential factors for the development of autonomous networks. ‘The network must be well monitored, with tools that provide visibility and observability. A good inventory is essential to know the existing assets and their relationships.Finally, the network must be based on market standards that allow configuration through open interfaces with languages designed for this purpose,’ he says.
Fuentes believes we are on the right track.‘For this to be feasible, it is essential that the data be of high quality and easily extractable. And I believe that this option exists in Spanish networks,’ he says.
The head of Ericsson says that ‘most network operators are at Level 2-3 (partial to conditional autonomy), according to the TM Forum (TMF) classification, where AI-based automation is used to optimise network operations, although human need is still necessary for the most critical functions’.
For example, it notes that autonomic network use cases such as predictive maintenance, anomaly detection and AI-based optimisation are already available in commercial deployments.
In addition, he highlights that ‘leading network operators are actively working on reaching Level 4 (high autonomy), with an approach based on declarative predicates (intents) and multi-domain closed-loop actuation solutions’.
In this sense, he anticipates that by 2025-2027, a majority adoption of Level 3-4 (conditional to high autonomy) capabilities is expected, ‘where AI will manage real-time network parameter adjustments for the optimisation of operators’ radio access networks, mobile network and transport network’.
The first Level 5 (full autonomy) networks deployed by industry leaders are expected to arrive in 2030. ‘AI will comprehensively manage end-to-end operations, based on intents, network self-healing capabilities and multi-domain orchestration, with minimal user oversight,’ says Muñiz.
Sánchez details some of the advances that are already a reality, such as ‘private 5G networks that automatically adjust to the demand of users in the required area, which is called self-configuration’, as well as ‘mobile networks that automatically reroute to avoid congestion (self-optimisation)’.
In addition, he notes that ‘since the introduction of 5G, the implementation of so-called network slicing, which allows companies and industrial sectors to use customised networks optimised for their specific needs, is becoming a growing reality’.
He also points out that ‘in the specific situation of emergency networks, the use of drones to re-establish communications in areas affected by natural disasters and their management from the network, when other network resources are disabled, is another example of real application of these autonomous networks’.
For his part, the head of Telefónica remarked that ‘network automation is not something for the future, but rather a gradual process that has been implemented for years’.
For example, he explains that the following are already common functionalities: ‘the automatic entry into operation of backup networks when a communications line goes down; root cause analysis of incidents, capable of understanding that 5,000 apparently different incidents have the same cause in a network element; or preventive analysis, capable of foreseeing that a service is going to have performance problems in the next few hours and acting automatically by applying preventive measures to avoid incidents’.
Looking to the future, he indicates that we will move towards the so-called Zero Touch, with networks ‘capable of self-diagnosing and correcting themselves automatically, without manual intervention of any kind, achieving 100% availability of services’, for which AI technologies applied to networks will be essential. ‘This allows us to dedicate resources to innovate and bring out new services for customers with greater agility,’ he says.
The advent of 6G, a new dimension
‘The convergence of autonomous networks and the future 6G will lead to fully self-managed networks, powered by native AI, with self-optimisation capabilities and real-time adaptability,’ Muñiz predicts.
‘Thanks to intent-based AI, 6G networks will enable hyper-personalised and on-demand services, dynamic network segmentation and predictive resource allocation for advanced applications such as holography, AI-based robotics and the massive internet of things. End-to-end closed-loop performance automation will seamlessly manage radio access networks, mobile network, edge network and cloud resources. Furthermore, through AI-driven digital twin technology, the network will undergo a continuous performance optimisation process, proactively anticipating failures. Additionally, through AI-based sustainable energy management techniques, the carbon footprint will be minimised and the cost-effectiveness of 6G networks will be maximised,’ he says.
He also notes that ‘autonomous networks and 6G will contribute to energy efficiency and sustainability goals, optimising resource use and reducing operational overhead’. In addition, he notes that ‘the ultra-low latency of 6G will enable real-time autonomous network operations, improving responsiveness and reliability’.
Furthermore, Marfil stresses that the standardisation that will come with the development of 6G ‘will bring the interoperability and economy of scale needed for the automation ecosystem to take off’. ‘The fact that it is part of a standard will mean, above all, interoperability between manufacturers from different vendors, so that, for example, AI systems implemented by some vendors can understand and process data generated by technologies from other vendors,’ he says.
What are companies doing?
Marfil explains that Telefónica has been introducing automation mechanisms in its networks for years, through an initiative called ‘Autonomous Network Journey’.
‘From big data, machine learning and deep learning technologies for the automatic analysis of incidents and preventive network maintenance, the use of DevOps technologies to automate and speed up the deployment of new network functionalities, to the use of generative AI to speed up the processing of customer requests made by email or voice messages, Telefónica’s objective in Spain is to reach a 3.7 index in 2026 on the recognised automation scale defined by the international organisation TM Forum, with the long-term goal of reaching total automation, for which the industry still needs to perfect and adapt AI technology a little more to the needs and particularities of Telco network operation,’ he specifies.
Gaubeca points out that Grupo Aire is making progress in the development of observability and asset inventory. ‘Without them, it would not be possible to get to the last phase,’ he says. ‘The more sensors there are, the better decisions an autonomous system can make, as it will be better able to have a view of what is happening. There is a long way to go,’ he says.
Ericsson has several examples of AI-driven network automation, intent-based operations and closed-loop actuation solutions in the telecoms industry.
‘For example, Ericsson Intelligent Automation Platform (EIAP) is an open platform that enables AI-based automation in the radio access network by running AI-powered rApps. These applications optimise radio network performance through anomaly detection, recommendation generation and automated action implementation,’ says Muñiz.
EIAP has been integrated into Snowflake’s AI Data Cloud platform. ‘The rApps developed on top of EIAP will benefit from access to richer data sets that combine data from operations support systems (OSS), business support systems (BSS) and third-party data found in the Snowflake Marketplace,’ Snowflake details.
‘This rApp leverages EIAP, multi-agent frameworks and LLM hosted on Snowflake to automate troubleshooting and RAN management. By integrating anomaly detection, KPI analysis and root cause analysis (RCA), it reduces mean time to resolution (MTTR), enables intent-based automation and drives self-optimising networks with AI data close to the network,’ he adds.
Muñiz points out that Ericsson has real-world deployments with telecom operators such as DNB Malaysia, where it has implemented the first operations using AI-driven, intent-based 5G slicing. ‘This solution has significantly improved the service level agreement (SLA), achieving 100% compliance for premium services and reducing the need for manual intervention,’ he explains.
In addition, he stresses that ‘Ericsson plays a key role in defining frameworks for autonomous networks at an industry level, actively contributing in TM Forum, 3GPP, O-RAN and ETSI’.
Nokia is also working in this direction. For example, by providing a common and reliable layer of data consumable by tools including AI to enable efficient management between equipment, as Rionegro points out.
Also by creating intent-based networks, ‘shifting the paradigm from “how” to “what”, allowing users to define desired outcomes rather than technical configurations’; or by deploying advanced capabilities, ‘implementing 360° observability, responsible use of AI and optimisation to discover and design new automation patterns’.
Risks of autonomous networks
While autonomous networks offer many advantages, they also carry certain risks. Some stem from the data that AI uses. ‘If biased or unrepresentative data is used, the models could perpetuate those biases. Therefore, it is necessary to carry out a detailed analysis of which data is collected, which is of real value to the desired objective, and which should be discarded. For example, if you only take into account residential uses, you will not be able to detect anomalies typical of business environments,’ says Fuentes.
Similarly, the DigitalES CEO warns that ‘AI algorithms can learn biases from the data they were trained on, which can lead to erroneous or unfair decisions in network traffic management’. Continuing with the example above, training a model with data that prioritises certain types of traffic can lead to an uneven distribution of bandwidth.
There is also the risk of AI hallucinations to consider. ‘This occurs when AI generates answers or makes decisions based on incorrect patterns, identifying non-existent correlations in the data. This can lead to failures in network optimisation, such as inefficient resource allocation or false alarms about cyber attacks,’ says Sánchez.
Cybersecurity is also a concern. ‘A poorly configured AI can become a point of vulnerability in the network. Attackers could manipulate the AI model with misleading data (adversarial attacks) to make bad decisions, such as allowing malicious traffic or blocking legitimate services,’ warns the DigitalES spokesperson.
For all these reasons, Fuentes believes that ‘it is advisable to monitor the model’s performance in real time and maintain a person to supervise and validate the most critical decisions’. He also stresses that ‘periodic monitoring and retraining, incorporating new data, allows the model to adjust to the variations and evolutions of the network’. He also insists that ‘transparency in the operation of the system facilitates the detection and resolution of faults’.
Sánchez also believes that ‘the way to mitigate risks is to design networks with hybrid control layers that combine AI and manual management, also for cybersecurity, establishing limits to the autonomy of AI and maintaining manual supervision’.
In addition, he points out that ‘it is essential to continuously monitor the results generated by AI and validate their accuracy, as well as to use the explainability of AI (XAI) to understand and justify the system’s decisions’. All this, without forgetting that ‘the “fuel” of all AI is data’, so it is necessary to use diverse and representative datasets for training models and to implement continuous audits and assessments of bias in the algorithms.
Other uses of AI in industry
Aside from autonomous networks, the telecoms sector is embracing AI for many other purposes. ‘Optimising customer service through intelligent chatbots and virtual assistants is already a reality. Progress will also be made in customer classification and, thanks to this, in the personalisation of offers and services based on predictive analysis of user behaviour,’ says the Aotec spokeswoman.
Sanchez notes that generative AI is being employed to generate automatic response scripts to cybersecurity incidents in real time. ‘These scripts can be designed to execute specific actions, such as isolating compromised devices, blocking unauthorised access or restoring affected systems, improving the efficiency and speed of incident response,’ he says.
Generative AI is also being used to simulate different cybersecurity scenarios, ‘allowing companies to anticipate and prepare for potential cyber attacks, as AI can generate models that assess the impact of various threats and vulnerabilities on the company’s digital infrastructure, providing a comprehensive view of potential risks and mitigation strategies,’ the DigitalES CEO stresses.
Another application is the generation of synthetic data for telecom application testing, mimicking network scenarios and user behaviour to test and validate telecom applications, such as network optimisation and failure analysis, without the need to collect real data. ‘It saves time and resources and ensures user privacy,’ explains Sánchez.
For his part, Marfil emphasises predictive systems. ‘Based on thousands of parameters, able to predict the load the network will have on each of its elements. This brings us such curious applications as predicting the optimal route for a drone to take to carry a package between two points, depending on which path offers the best connectivity at the specific time of the flight; or deciding which route a robot transporting a part in a factory should follow, depending on the placement of the thousands of connected devices and the use they will be making of telecommunications,’ he explains.
And AI can also be of great use to telecoms operators in business and marketing-related applications. For example, Snowflake highlights the use of AI-powered applications to prevent fraud, non-payment and churn by exploiting their data to predict the likelihood of non-payment, anticipate customer complaints related to billing or assess fraud risk in real time.
Similarly, telcos can use AI to mine customer data, from billing and subscription information to streaming and usage data, to create personalised marketing campaigns. ‘For example, a telco can identify customers with high roaming data usage who could benefit from a better, enhanced international plan,’ notes Snoflake.
AI can also be used to segment audiences, exclude customers with open service cases, test different marketing channels, measure which channels are most effective in driving upgrades, and so on.