What is The Environmental Impact of the Development of Generative AI?
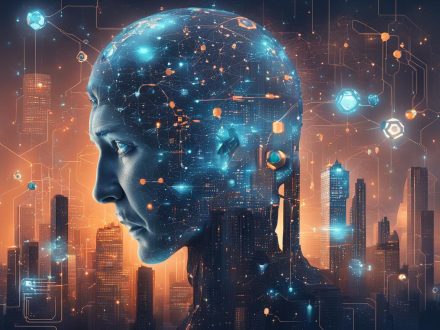
The new era of generative AI is opening up a whole new world of possibilities, but it also forces us to question the environmental consequences.
The explosion of generative AI in recent years has been spectacular. New and surprising applications for this technology are found almost every day, and it is spreading to all sectors of activity, democratising its use.
Moreover, generative AI has led to the visibilisation of AI, a technology that previously remained in the ‘back room’. Users used to benefit from recommendations from streaming services and ecommerces or used smart speakers, for example, but lacked the option to use AI first-hand.
However, generative AI allows the user to take control and use this technology for any purpose: writing an email, searching for information on a certain topic, generating an image, preparing a presentation…
If the rise of AI was already causing a significant increase in computing and data transmission needs, considering the environmental impact it entails, as we saw in this report, the new era being inaugurated with generative AI is triggering concerns about the sustainability of its advancement.
‘We are at a time of AI explosion, in which generative AI plays a prominent role, as it is the most widely used by the end user. AI requires greater hardware resources in datacentres with even greater computational capacity. This means that more powerful GPUs are constantly being developed, which boost the thermal design power of processors, yet also double their energy consumption, potentially increasing carbon emissions by up to 30%,” said Matias Sosa, cloud specialist & product marketing manager at OVHcloud.
‘We are facing a collective challenge, which requires a multidimensional, industry-wide approach. This involves not only optimising cloud infrastructure, but also rethinking the design of AI models, with redesigned software that requires less training and computer resources,” he adds.
In this sense, the appearance of DeepSeek in the generative AI arena may be a turning point, as it appears to be able to do the same as ChatGPT’s more advanced models, but with far fewer resources.
Furthermore, Sosa believes that ‘performance optimisation must go hand in hand with energy optimisation’. ‘The adoption of a circular economy, focusing on the reuse and refurbishment of equipment, is also a key factor, which can benefit the entire industry. Hardware and software vendors must strive to be more efficient in our design, developing solutions that optimise data consumption and deploying more energy-efficient, renewable energy-based data centres. This will enable customers to also reduce the carbon footprint of their IT services,’ he says.
Energy and water consumption
One of the biggest concerns about the rise of generative AI is the high energy consumption that comes with it. ‘Energy consumption is growing exponentially. According to our recently published report on the sustainability of generative AI, global electricity demand for data centres is expected to more than double from 460 TWh in 2022 to 1,000 TWh in 2026, driven mainly by generative AI,’ specifies Manuel Cid, vice president and head of Insight & Data at Capgemini in Spain.
For example, he points out that ‘training a GPT-4 model (1.76 trillion parameters) consumes between 51,772 and 62,319 MWh, equivalent to the annual consumption of 5,000 US households’.
Generative AI also generates significant water consumption. According to a recent study by researchers at the universities of California and Texas, training the large language model (LLM) GPT-3 can consume 700,000 litres of water to cool the servers. And global demand for AI is expected to draw 4.2 to 6.6 billion cubic metres of water by 2027.
Cid also notes that ‘it is estimated that running 20 to 50 queries on an LLM requires approximately 500 millilitres of water at a time’. The same amount of water consumed to resolve a request as simple as writing a 100-word email in ChatGPT, according to research by the University of California, as reported in The Washington Post.
Similarly, Federico Vadillo, senior solutions engineer at Akamai, points out that ‘an average-sized data centre can consume up to 300,000 gallons of water (1.1 million litres) per day for cooling’.
In addition, the Capgemini manager points out that ‘the manufacturing of microchips used in AI consumes around 8,328 litres of ultrapure water per unit’.
More CO2 emissions and e-waste
Another pernicious consequence of the advance of generative AI is the increase in greenhouse gas emissions. ‘In terms of CO2 emissions, the carbon footprint associated with the training of large models such as GPT-4 is equivalent to the electricity consumption of thousands of households for a year,’ notes Cid.
Similarly, Vadillo notes that ‘the training of a single AI model can generate more than 626,000 pounds (284,000 kilograms) of CO2, equivalent to the emissions of five cars over its lifetime,’ says Vadillo.
In addition, the boom in generative AI is leading to an increase in e-waste. In relation to e-waste, it is estimated that generative AI could generate between 1.2 and 5 million metric tons of e-waste by 2030,’ says the Capgemini spokesperson.
Similarly, the Akamai representative indicates that ‘a 1,000-fold increase in AI-related e-waste is expected by 2030’.
Does the use of generative AI pay off?
With all this data in hand, the question arises as to whether it is really worth using generative AI. It is clear that the use of this technology improves performance and multiplies productivity, but it is worth considering whether it is worth the price we pay for it.
‘There is no doubt that generative AI has brought many benefits, not least the positive impact on task automation, allowing many professionals to focus on higher-value activities and thus increase business productivity. However, it should be noted that the constant training and deployment of generative AI platforms consumes a lot of indirect energy, constantly demanding resources,’ acknowledges Sosa.
‘Currently, we may be at a point of imbalance, as the effects on energy impact are high. But we are also seeing that there is a search for energy efficiency to ensure the sustainability of these use cases and business models. The optimisation of models could help a lot here, with specialised models by sector of activity. For example, in sectors such as logistics or industry, AI models could emerge for the automation of specific tasks. As they are more tailored to specific uses, they would require less processing resources and thus less energy resources, with a considerable increase in productivity,’ he says.
For his part, David Blázquez, Head of Institutional Relations for Infrastructure and Energy at AWS in Spain and Portugal, considers that ‘the balance is positive’. ‘Generative AI is one of the most transformative technologies of our time, addressing some of humanity’s most complex problems, increasing performance and maximising productivity. Generative AI will reinvent all customer experiences and applications and is already driving efficiency for people and organisations,’ he says.
And he provides some data that reinforces his position. ‘According to a study by Access Partnership in collaboration with AWS, employee productivity could increase by 45% through the adoption of AI or generative AI. In addition, companies using the AWS cloud can reduce their carbon footprint by up to 99% by optimising their workloads. These are just a few examples of the positive impact on productivity and carbon footprint reduction that AI and generative AI can have on organisations.’
On the other hand, he remarks that ‘investment in new data centres that support AI growth, such as the already operational AWS region in Aragon, which will represent an investment of €15.7 billion in Spain, generates new opportunities and skilled jobs for local communities’.
‘In fact, in a country like Spain, which last year generated more energy than it consumed, the proliferation of data centres and the rise of AI is a great opportunity to accelerate digital transformation and become an international benchmark for innovation,’ he emphasises.
Equinix also points out that ‘once responsibly designed and deployed, AI solutions have the potential to increase productivity and operational efficiency, while reducing energy and environmental costs in the long term’.
Similarly, Cid says, ‘Generative AI has great potential to improve efficiency and address multiple challenges in diverse industries and sectors in a much more productive way’.
‘These advantages represent significant opportunities for organisations looking to accelerate their transition to more sustainable models. While the current environmental impact of generative AI is notable, there are tools and strategies available, such as the use of smaller models, optimisation through quantization and compression techniques, and leveraging sustainable infrastructures, that can mitigate these effects. With the right implementation, generative AI can deliver a positive bottom line in terms of productivity,’ he says.
However, Akamai acknowledges that it is not easy to pinpoint which way the balance tips when weighing up the positive and negative impacts. ‘More research is needed to accurately quantify the benefits and costs,’ he says. He believes, however, that ‘it has the potential to contribute significantly to sustainability initiatives if used responsibly’.
Towards more responsible use
That is precisely the key: the responsible use of technology. However, we must ask ourselves whether companies are aware of the environmental footprint that comes with the use of generative AI.
‘Unfortunately, most companies are either not fully aware or have chosen to overlook it for quite some time. Only 12% measure the environmental impact of generative AI. And less than 20% consider it a key factor when selecting models. We are now starting to see organisations incorporating sustainability measures into the AI lifecycle, with 31% of executives saying their organisations have already taken steps in this direction,’ said the Capgemini executive.
In fact, environmental criteria still play little role in companies’ decisions when it comes to choosing a vendor or building generative AI models. The Akamai expert points out that ‘only one in five companies currently consider environmental footprint as a primary factor when selecting AI models,’ according to a PwC study. However, 70% of companies adopting generative AI by 2027 are projected to rank sustainability as one of their top selection criteria, according to a Gartner report.
Similarly, Cid notes that ‘currently, only 15% of companies consider sustainability as a key criterion when selecting generative AI vendors, highlighting a limited focus on this aspect of decision-making’.
However, he says that ‘the mindset is changing rapidly, with 55% of executives saying that including sustainability as a key factor in vendor selection could significantly reduce the environmental footprint of this technology’.
‘This shift is particularly relevant in a context where both customers and regulations are putting increasing pressure on companies to adopt more responsible practices,’ he says.
Similarly, the OVHcloud representative insists that we are not only facing an environmental challenge, but also a regulatory one, as we have to comply with the sustainability goals of the 2030 Agenda. ‘Many organisations are starting to ask for this information in order to comply with regulations, and it is even an increasingly decisive factor for accessing investment funds. This will contribute to it becoming a very relevant factor in the medium term,’ he predicts.
In any case, the Capgemini spokesperson reminds us that there are already options that can guide organisations in this direction. ‘Tools such as green data centres, the use of efficient hardware and the selection of locations with a lower environmental impact for servers – for example, in regions with a higher proportion of renewable energy – can make a significant difference to the environmental footprint of generative AI operations. This approach not only allows companies to align with regulatory objectives and consumer expectations, but also offers opportunities to optimise operating costs through sustainable practices.
Suppliers take the lead
For companies to start taking sustainability into account when making decisions around generative AI, they first need to become aware of the current situation.
‘More education on the responsible use of this technology is needed to mitigate its negative impacts,’ says Vadillo. Equinix also believes that ‘training and expert advice are key for companies to understand the benefits of AI and, at the same time, deploy their infrastructure in sustainable spaces’.
This requires vendor involvement. Blázquez details some of the tools that AWS offers, such as the AWS Well-Architected Framework, which helps its customers understand the impact of the decisions they make when building workloads on AWS.
It also has the Customer Carbon Footprint Tool, which allows its customers to measure and forecast the estimated carbon emissions from the use of AWS services. ‘They can improve their understanding of the factors driving their carbon footprint, from the services they use to the AWS regions where they host their data, and predict how their emissions will evolve in their transition to sustainability as Amazon moves forward with implementing its renewable energy programmes,’ he says.
Vadillo notes that Akamai has also developed a carbon calculator so that its customers can measure the emissions associated with their use of the Akamai Connected Cloud. And so has OVHcloud. ‘It provides customers with transparent and comprehensive information on scopes 1, 2, 3 of their carbon footprint, from manufacturing to infrastructure operation,’ says Sosa.
The OVHcloud manager stresses that the development of carbon measurement tools ‘gives users a complete and accurate view of their environmental impact, as part of a proactive approach to raise awareness and transition in favour of reducing their footprint’.
‘The future of AI relies on close collaboration between cloud providers, AI developers, end users and policymakers to create innovative and sustainable solutions. This transparency and capacity for collective innovation is essential in the transition to sustainable AI, aligned with energy efficiency and responsible technological innovation,’ he adds.
But it is not enough just for organisations to raise awareness; suppliers must also take the initiative to reduce the environmental impact of using these technologies. And they are doing just that.
‘Our measures include investing in energy-efficient chips, such as AWS Trainium and Graviton; innovation in cooling technologies, such as direct-to-chip configurable liquid cooling; optimising the design of our data centres to maximise energy use and minimise losses; using 100% renewable energy in our data centres, such as those in Aragon; renewable energy projects, such as those we have planned in Spain; and a commitment to return more water to the community than we use,’ Blázquez explains.
‘Our innovations in energy efficiency, such as new cooling technologies and the use of purpose-built chips, such as AWS Trainium and Inferentia, allow us to deliver 12% more computing power per site with less environmental impact. And our infrastructure is up to 4.1 times more energy efficient than local facilities. In addition, improvements in the design of our data centres, such as simplifying electrical distribution and mechanical systems, help us reduce overall energy consumption and minimise the risk of failures,’ he adds.
In terms of water consumption, it has developed mechanical cooling solutions that provide configurable direct-to-chip cooling in both its existing and new data centres, minimising water usage per megawatt. It has also set a target of returning more water to the community than its data centres use by 2030.
In terms of CO2 emissions, it points out that its data centres in Aragon have been 100% powered by renewable energy since the start of operations. He also notes that AWS and Amazon are investing in 79 solar and wind energy projects in Spain that will add more than 2.9 GW of clean energy to the grid.
Vadillo also outlines the measures deployed by Akamai, such as its goal of achieving net zero emissions and a zero carbon platform by 2030, its efforts to increase the energy efficiency of its network by 30% annually or the processing of 100% of its e-waste through e-Stewards certified suppliers, in addition to the development of its carbon calculator.
Equinix has also set itself the goal of achieving climate neutrality by 2030, ‘through science-based commitments’, he says. ‘Aware that cooling represents one of the main energy challenges, we have implemented high-efficiency technologies, such as advanced liquid cooling solutions, to handle high-density workloads in a more sustainable way,’ he stresses.
‘ In parallel, we drive the circular economy throughout the entire lifecycle of our data centres, working with local communities to minimise resource consumption and waste. In addition, we were the first operator in the sector to join the European Pact for Climate Neutrality in data centres, operating our facilities at temperatures close to 27°C,’ he says.
He also points out that Equinix is supplied with 100% renewable energy in Europe, closing purchase agreements to promote the generation of clean energy.
Sosa also emphasises that OVHcloud developed a liquid cooling system more than 20 years ago to dissipate the heat emitted by the most energy-consuming components of the servers, the CPU and GPU processors. ‘This allows us to reduce the energy costs of our 43 datacentres, with some of the best usage rates in the industry: we have a PUE (Power Usage Effectiveness) of 1.26 and a WUE (Water Usage Effectiveness) of 0.37 L/kWh,’ he specifies.
He also emphasises that the company has an industrial model that allows it to apply the circular economy. ‘We dismantle 100% of the servers after use, providing components for reuse and extending their useful life to at least 5 years. These circular economy principles are also applied throughout our supply chain, including the refurbishment of old buildings to house our data centres. Wherever possible, we try to reuse existing facilities. In fact, 28 of our datacentres are in ‘recycled’ buildings, eliminating unnecessary carbon emissions associated with construction,’ he says.
What role do AIPCs play?
The development of AI-optimised computers, so-called AIPCs, can also help reduce the need for data centre processing capacity.
‘They have the potential to absorb some of the processing load needed for AI tasks, which could help spread the energy demand. However, it is important to consider that these devices also consume energy and resources. Their net impact on sustainability will depend on how they are deployed and used,’ notes the Akamai official.
Equinix notes that ‘AIPCs or analogue formats are becoming relevant for inference and training processes, offering lower latencies and greater efficiency to complement data centres.
In any case, OVHcloud considers AIPCs to still represent’ a segment with minimal impact, with very specific uses for working with data locally or dedicated to initial model training or AI pre-processing tasks’.
‘The main advantage is that they avoid the costs and energy impact derived from data transfer, but when it comes to scaling and demanding more processing capacity, costs and energy consumption can increase significantly, leaving them at a clear disadvantage compared to the cloud,’ he says.
For his part, Cid recalls that ‘technologies such as Nvidia Blackwell chips and AWS Trainium have significantly reduced power consumption, allowing data to be processed more efficiently and at a much lower operating cost’.
In addition, he says, ‘the adoption of these technologies is also complemented by model parallelism techniques’. ‘Platforms such as AWS SageMaker allow distributed training to be run and models to be processed more efficiently across multiple graphics processing units (GPUs). This optimises the utilisation of available resources, reduces training time and minimises the energy used to complete the process,’ he said.
Equinix also notes that it is collaborating with partners such as Dell Technologies and Nvidia to provide private AI solutions that enable training and model execution in a highly scalable and energy-efficient manner. These solutions maintain data control and support enterprises’ sustainability goals.
‘To this end, we develop AI-ready datacentres with high energy density and efficient cooling systems. By placing specialised computing close to the data sources, organisations benefit from the advantages of private AI, such as lower latency, increased security and the high performance demanded by the most demanding use cases,’ he says.